Machine learning improves predictions of agricultural nitrous oxide emissions
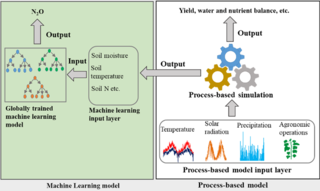
Researchers have developed a new tool that can significantly increase the accuracy of nitrous oxide emission predictions, especially when coupled with a conventional soil nitrogen model.
The Science
Great Lakes Bioenergy Research Center (GLBRC) scientists have established a machine learning model to help explain variation in nitrous oxide emissions from agricultural soils. Nitrous oxide is a naturally occurring greenhouse gas 300 times more potent than carbon dioxide, and agriculture is a major global source. Trained on data collected from GLBRC field sites in Michigan and Wisconsin, the tool can predict nitrous oxide fluxes much more accurately than existing models. When coupled with a conventional soil nitrogen model, the new machine learning tool improved the accuracy of predicted emissions by 2-3 fold relative to existing process-based models.
The Impact
This new approach establishes the potential for using machine learning to better predict greenhouse gas fluxes from diverse ecosystems, and provides a clear path for better representing nitrous oxide fluxes in global models that predict land-atmosphere interactions. The approach can also aid scientists developing strategies – including the use of bioenergy crops and other practices – to mitigate these emissions.
Summary
Nitrous oxide is a potent greenhouse gas that is accumulating in the atmosphere at unprecedented rates, largely due to agricultural management. However, nitrous oxide emissions are highly variable and irregular, making them hard to quantify accurately over large timeframes and geographical areas. GLBRC scientists created a machine learning model that more accurately predicts emissions with relatively few data inputs. The researchers used thousands of measured nitrous oxide fluxes from GLBRC cropping systems to train a model that can explain much of the variance that occurs in annual cropping systems. When combined with a conventional process-based model that predicts soil nitrogen availability, the machine learning model explained more than 50 percent of weekly flux variability in cropping systems not used to train the model, as compared to less than 20 percent accuracy for conventional nitrous oxide models. Training the model with data from other cropping systems, soils, climates, and management practices will further improve its predictive capacity.
Program Manager
N. Kent Peters
Program Manager, Office of Biological and Environmental Research
kent.peters@science.doe.gov, 301-903-5549
Corresponding Author
G. Philip Robertson
University Distinguished Professor, Michigan State University
robert30@msu.edu, 269-760-8364
Funding
Financial support was provided by the Great Lakes Bioenergy Research Center, U.S. Department of Energy, Office of Science, Office of Biological and Environmental Research (Award DE-SC0018409), by the National Science Foundation Long-term Ecological Research Program (DEB 1832042), by the USDA Long-term Agroecosystem Research Program, and by Michigan State University AgBioResearch.
Publication
Saha, D., Basso, B, and Robertson, G.P., “Machine learning improves predictions of agricultural nitrous oxide (N2O) emissions from intensively managed cropping systems,” Environmental Research Letters 16, 2 (2021). [DOI: 10.1088/1748-9326/abd2f3]
Related Links
https://iopscience.iop.org/article/10.1088/1748-9326/abd2f3