Bayesian experimental design identifies green solvents for bioproduct separation
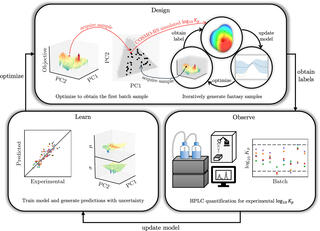
Background/Objective
Liquid–liquid extraction (LLE) is a commonly used technique for separation and purification of liquid-phase products with applications in industries including pharmaceuticals, petrochemicals, and renewable chemistry. Selection of appropriate solvents is a critical step in the design of LLE processes. This is especially challenging when selecting systems capable of separating multiple products while also balancing separation efficiency, selectivity, and environmental impact.
Approach
Scientists with the Great Lakes Bioenergy Research Center developed a Bayesian experimental design (BED) framework to guide solvent selection by predicting product partition coefficients (log Kp values) as thermodynamic parameters. The iterative approach combines Bayesian optimization with empirical measurements and leverages conductor-like screening model for realistic solvents (COSMO-RS) calculations to guide high-throughput experimentation capabilities.
Results
Using the design of solvent systems for separation of five lignin-derived aromatic products — para-hydroxybenzoic acid, syringaldehyde, syringic acid, vanillic acid, and vanillin — via centrifugal partition chromatography as a case study, researchers showed that within seven iterations the framework identified several green solvent mixtures that align with the desired criteria. The approach demonstrated significantly lower mean absolute error values than COSMO-RS alone with additional improvements in each iteration.
Impact
Results demonstrate the efficacy of the BED framework in optimizing green solvents for complex separations and highlight the potential to advance the field of green chemistry and develop sustainable industrial practices. The approach can be generalized to identify solvent systems for different separations challenges, and has potential for further integration with technoeconomic analysis and life cycle assessment to further optimize solvent design.