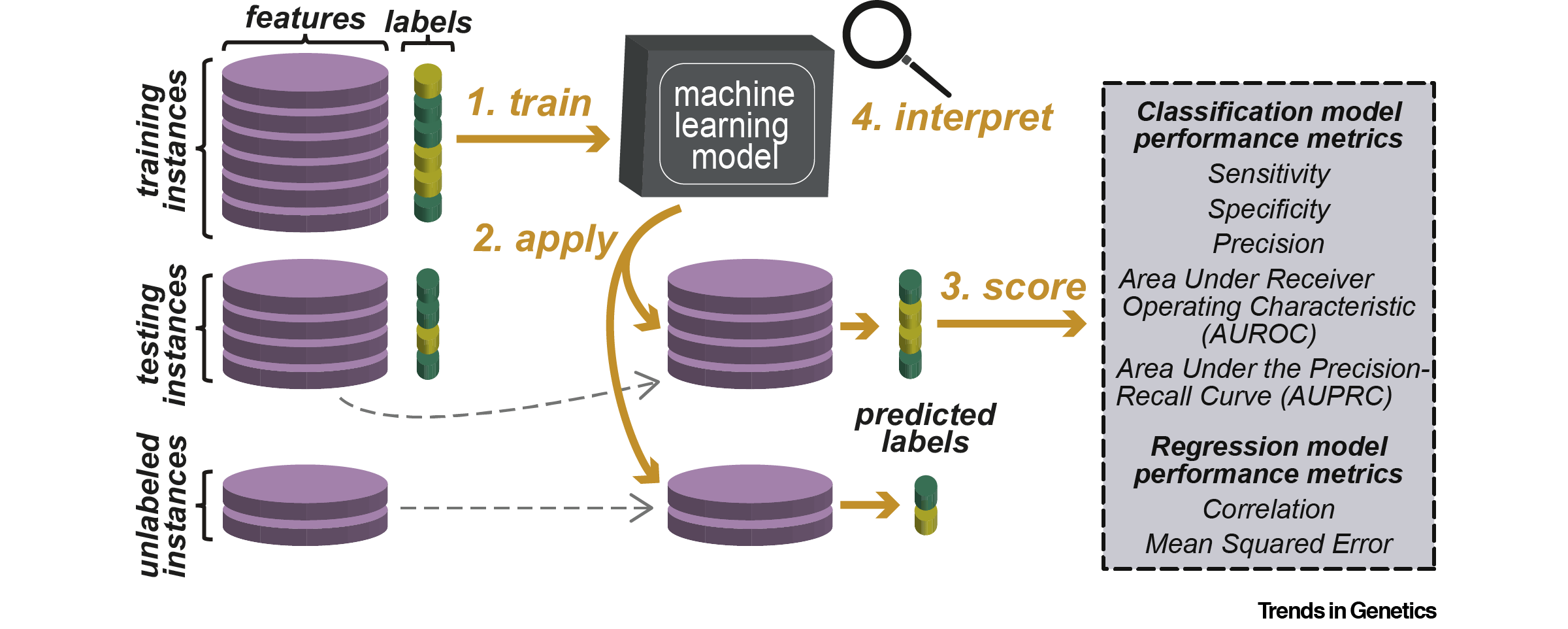
In a review published online April 17 in Trends in Genetics, “Opening the Black Box: Interpretable Machine Learning for Geneticists,” Shin-Han Shiu and his colleagues describe the power of machine learning tools for analyzing complex data and how these models can be applied to research questions in genetics and genomics.
Along with an overview of classical and deep-learning machine learning algorithms, the article discusses interpretation strategies to probe a trained model to better understand why and how the model works. Such strategies can provide insights into the underlying biology, such as relevant DNA motifs or microRNA features that best predict the expression level of a gene.
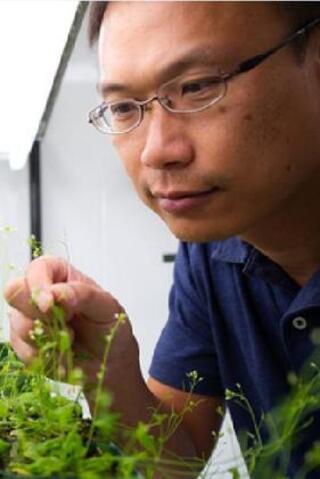
Shiu, a professor of plant biology and computational mathematics, science and engineering at Michigan State University, answered a few questions about the work.
What do you think your GLBRC colleagues would be most interested in knowing about machine learning and its use in genetics research?
Machine learning is an established field in computer science and is playing an increasingly important role in biological research over the past decade. Machine learning excels at finding patterns, including not very obvious ones, from a large set of data. With genetic data such as genome sequences accumulating at a rapid pace, these data can be integrated with machine learning approaches to answer challenging and/or new questions.
How are you using machine learning tools in your own work?
We have used machine learning tools to predict morphological and biochemical traits of switchgrass using genetic variation data, in collaboration with Michael Casler (UW–Madison) and David Lowry (MSU). Using the same set of tools, we are also collaborating with Sarah Evans at MSU on predicting below-ground microbiome diversity and with Audrey Gasch at UW–Madison on predicting the fitness of yeast strains overexpressing different genes for field-to-product integration purposes.
What do you see as some of the most exciting potential uses of machine learning in genetics and genomics research?
Because of its ability to deal with data from very different sources, machine learning can integrate heterogeneous data sets to provide better solutions to existing problems or problems that one cannot address due to the challenges in data integration. It has the potential to help us establish comprehensive models of, for example, how different genes, transcripts, and proteins impact cellular function; how genotypes are connected with phenotypes; or how genetic and environmental factors — biotic or abiotic — jointly impact plant growth and function.
Read more:
Christina B. Azodi, Jiliang Tang, and Shin-Han Shiu. “Opening the Black Box: Interpretable Machine Learning for Geneticists.” Trends in Genetics. Published online April 17, 2020. doi: https://doi.org/10.1016/j.tig.2020.03.005.