Modeled surface-atmosphere fluxes from paired sites in the Upper Great Lakes Region using neural networks
D.E. Reed et al. "Modeled surface-atmosphere fluxes from paired sites in the Upper Great Lakes Region using neural networks" JGR Biogeosciences 126, 8 (2021) [DOI: 10.1029/2021JG006363]
Measuring terrestrial carbon cycling is an important step in solving the global missing carbon sink budget, but direct measurements of carbon cycling are time intensive and costly. Eddy covariance methods are a commonly used technique to measure carbon cycling at scales of ∼1 km2 and efforts to scale these measurements to the entire landscape would allow a better comparison of carbon measurements of global models. Here we use a long-term record of carbon cycling from six paired sites plus a seventh reference site that are all spatially close to each other, with an average of ∼11 km separation. The goal is to use field observations from one of the paired sites, along with observations from the second site that can be measured easily, in order to estimate carbon cycling at the second site using artificial intelligence methods. We find that carbon cycling can be estimated with the same amount of uncertainty as the observations themselves. This is also true when using non-paired sites, for example, information from a corn site can model carbon fluxes at a grassland, and shows we could be able to extrapolate our understanding of carbon cycling much farther from a single measurement location.
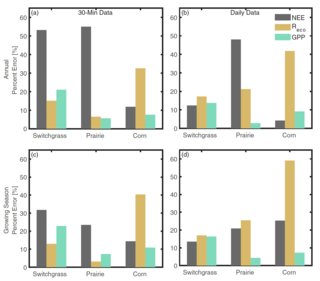