Heuristic computational model for predicting lignin solubility in tailored organic solvents
Z. Sumer and R.C. Van Lehn "Heuristic computational model for predicting lignin solubility in tailored organic solvents" ACS Sustainable Chemistry & Engineering 11:187-198 (2023) [DOI:10.1021/acssuschemeng.2c05199]
Lignin is a random heteropolymer that has been extensively studied as a renewable source of aromatic precursors for high-value chemicals, biofuels, and bioplastics. A key challenge in lignin valorization is the structural and compositional heterogeneity of lignin feedstocks. Solvent-based approaches are commonly used to fractionate lignin to reduce this heterogeneity, but solvent selection can be challenging due to variability in lignin composition. In this work, we developed computational methods to predict good and poor organic solvents as a function of lignin composition. We analyzed 28 different linear pentamer structures, 18 from known libraries and 10 hypothetical polymers, and calculated their activity coefficients in 50 different organic solvents by using the conductor-like screening model for realistic solvents. We used these data to train a regression model that enabled the extensive investigation of the impact of solvent and monolignol compositions on predicted lignin solubility. The exhaustive exploration of solubility trends using model predictions revealed sets of solvents, identified using Kamlet–Taft parameters, that are predicted to promote lignin dissolution regardless of lignin composition. We further identified solvents expected to selectively isolate lignin fractions enriched in certain subunits. These results establish heuristic guidelines for solvent selection that can be used to tailor fractionation processes for lignin feedstocks of distinct composition or to design new processes that isolate fractions with higher proportions of selected subunits.
Trained regression models are available at GitHub
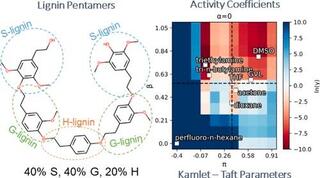